AI/ML applied to detecting liveness of online users
Mike Simpson
2nd May, 2022 4 min read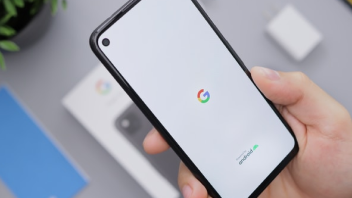
Biometrics are becoming the norm for authenticating online users, delivering a step change in user experience and improved authentication security. The security of biometric authentication is dependent on 2 factors – the ability of the system to match the authenticating user to their enrolled biometric (and not match anyone else), and the ability of the system to determine that the authenticating user is live and present, thereby mitigating the risk of fraudulent presentation attacks such as the use of photos, videos, masks, and deep fakes of the real user. This is often referred to as the ‘liveness’ of the user. Artificial Intelligence (AI) and Machine Learning (ML) models have made significant advances in recent years in detecting a wide range of presentation attacks. This post discusses the need for liveness, how it works, and approaches employed to discriminate between authentic live individuals and fraudulent presentation attacks.
What is ‘Liveness’
Face biometrics are gaining rapid acceptance with consumers and businesses as a convenient and secure method of identity verification. Face authentication can close security gaps in solutions that rely on something that can be lost or stolen, such as a password, an answer to a secret question, or SIM card credentials. Face recognition technology has advanced significantly over the last decade with advancements in artificial intelligence and the ubiquity of mobile phones with high resolution cameras.
The growing popularity of facial biometrics raises the question of security. If a potential fraudster can find an image of a person’s face does this create a security flaw in this method of authentication? To gain mainstream adoption, face authentication must be robust in distinguishing between a genuine live face and an attempt to spoof the system with a fraudulent presentation of a face. Automated detection of presentation attacks, where a trusted human is not supervising the authentication attempt, is a critical component of any facial authentication system.
The role of ML
Sophisticated machine learning algorithms such as Convolutional Neural Networks and Deep Neural Networks can now be implemented in large commercial authentication systems and deliver near-instantaneous results.
But how does it work?
Historically, the design of a liveness detection model required a deep understanding of the different types of presentation attack and how these attacks translate into different features within images. For example, if a fraudulent actor is presenting a photo or video from their mobile device, the ML model will need to be trained to search for features within the image such as borders of a phone, or glare from the screen of the playback device.
Since there are a wide range of potential presentation attacks, some features might work well for one class of attacks, and work poorly for others. To increase the quality of the liveness detection model, it might seem logical to combine as many different features and modeling approaches as possible. But this can also lead to higher false positives (live people predicted as fake).
Deep learning-based methods have significantly improve the quality of liveness detectors. Convolutional Neural Networks (CNN) can extract the important features from an image, and ‘backpropagation’ forces the network to learn which parts of an image is important to classify the input sample as live or as a spoof. The more images (both live and fake) available to train the CNN, the higher the accuracy of the system. The extracted features are more robust changes in contextual factors such as illumination and image quality.
Passive vs. Active Liveness
Many of today’s facial liveness technologies are “active”. This means the user is asked to respond to a ‘challenge’ which has some level of randomness. For example, the user may be asked to blink, or turn their heads in a specific direction. The purpose of the random challenge is to mitigate the risk of specific presentation attacks such as photo and video playback attacks. In contrast, “passive” liveness tests do not present any challenges to the user, or if they do, those challenges are imperceptible to the user. The experience for the user is the same as taking a “selfie”. Some commercial services combine both passive and active liveness tests, with the active test invoked if a specific threshold of liveness confidence is not attained with the passive test.
While different liveness service providers have different approaches and focus on different features, the goal for each liveness service is the same – deliver the lowest possible False Accept Rate (accepting an imposter) and the lowest possible False Reject Rate (denying the real live user), while also delivering a quick and seamless user experience.
Biopass + Liveness
Next article
truuth launches EU platform for GDPR compliant identity verification
Biometrics are becoming the norm for authenticating online users, delivering a step change in user experience and improved authentication security. The security of biometric authentication.