Should we be worried about Deepfake IDs?
Mike Simpson
7th November, 2023 5 min read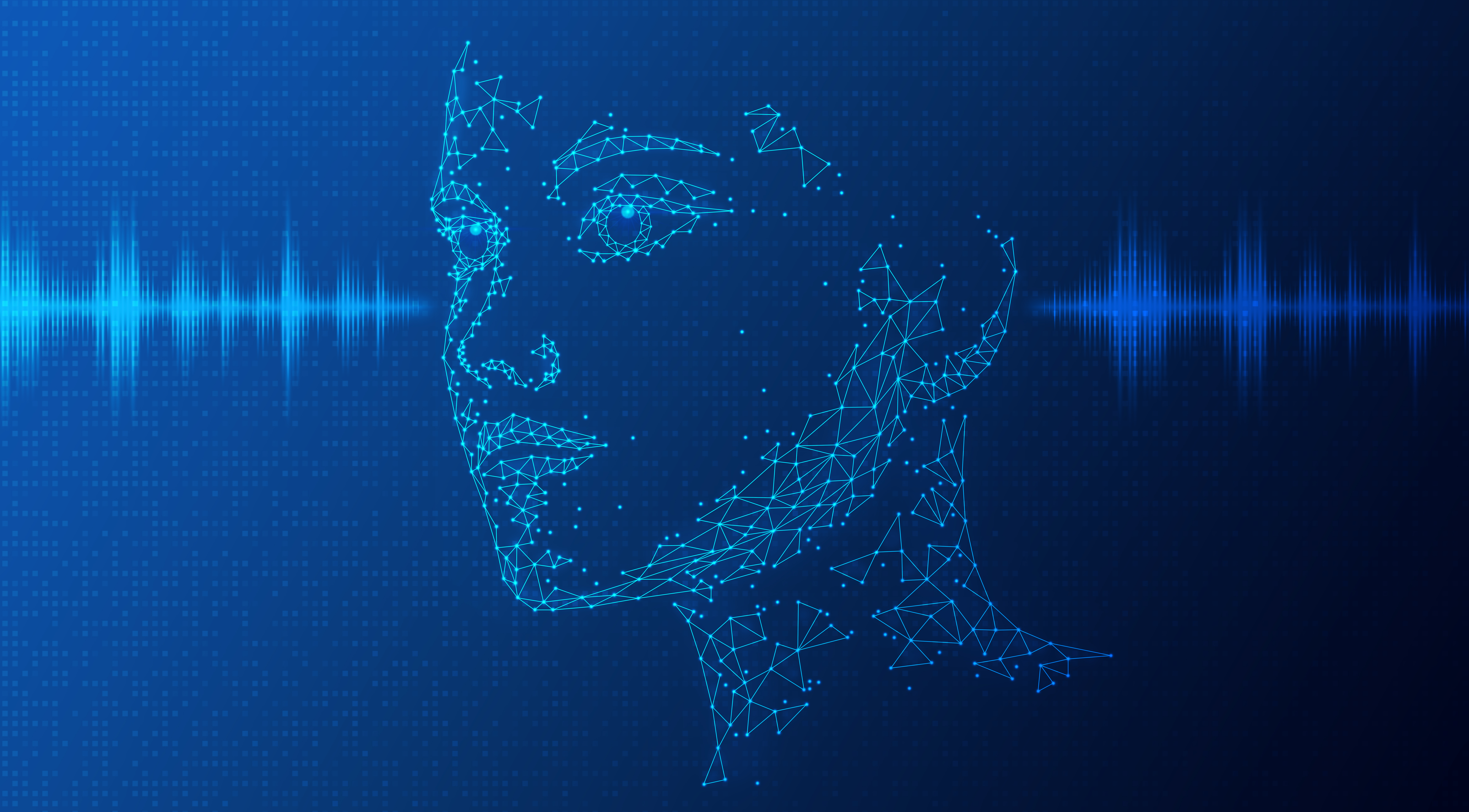
Deepfakes are an emergent threat falling under the greater and more pervasive umbrella of synthetic identities. They utilize a form of artificial intelligence/machine learning (AI/ML) to build believable, realistic videos, pictures, audio, to text to create new fictitious identities. Fraudulent actors are using these deepfakes to create new accounts with banks, telcos, utilities and a wide variety of online businesses. No sector is immune and the threat level is rising with each month. In September 2023, deepfake IDs accounted for 5% of all customer applications for one of our clients.
Background on Deepfakes
Deepfakes are a subset of the general category of “synthetic content” which can be broadly defined as any media which has been created or modified through the use of artificial intelligence/machine learning (AI/ML).
Since the first deepfake in 2017, there have been many developments in deepfake technologies. The AI/ML models enable the ‘swapping’ of the face of one person onto another person’s face and body. This is done using Encoder or Deep Neural Network (DNN) technology to create a face swap. To train the face swap model using an autoencoder, preprocessed samples of person A and person B are mapped to the same intermediate compressed latent space using the same encoder parameters. Once the networks are trained the target video (or image) of A is fed frame by frame into the common encoder network, and then decoded by person B’s decoder network.
There are many applications that allow a user to swap faces but not all use the same technology including FaceShifter, FaceSwap, DeepFace Lab, Reface, and TikTok. Apps like Snapchat and TikTok offer dramatically lowered computational and expertise requirements that allow users to generate various manipulations in real time.
The Role of GANs
A key technology leveraged to produce deepfakes and other synthetic media is the concept of a “Generative Adversarial Network” or GAN. In a GAN, two machine learning networks are utilized to develop synthetic content through an adversarial process. The first network is the “generator.” Data that represents the type of content to be created is fed to this first network so that it can ‘learn’ the characteristics of that type of data. The generator then attempts to create new examples of that data which exhibit the same characteristics of the original data. These generated examples are then presented to the second machine learning network, which has also been trained (but through a slightly different approach) to ‘learn’ to identify the characteristics of that type of data. This second network (the “adversary”) attempts to detect flaws in the presented examples and rejects those which it determines do not exhibit the same sort of characteristics as the original data – identifying them as “fakes.” These fakes are then ‘returned’ to the first network, so it can learn to improve its process of creating new data. This back and forth continues until the generator produces fake content that the adversary identifies as real. While human faces are a popular subject of GANs, they can be applied to any content. The more detailed (i.e., realistic) the content used to train the networks in a GAN, the more realistic the output will be.
The Threat of Deepfakes
A recent report from US Homeland Security states that:
“Malign actors associated with nation-states, including Russia and China, have conducted influence operations leveraging GAN-generated images on their social media profiles. They used these synthetic personas to build credibility and believability to promote a localized or regional issue. This is not a singular incident and it seems to be a common technique now in the age of influence campaigns.”
Deepfakes pose a threat for individuals and industries, including potential largescale impacts to nations, governments, businesses, and society. Social media disinformation campaigns are operated at scale by well-funded nation state actors. Experts agree that the technology is rapidly advancing, and the cost of producing top-quality deepfake content is declining. As a result, the threat landscape is evolving rapidly and attacks will become easier and more successful, and the efforts to counter and mitigate these threats will need orchestration and collaboration by governments, industry, and society.
Threat Scenarios
The Homeland Security Report “Increasing Threat of Deepfake Identities” describes a range of threat scenarios resulting from deepfakes. The scenarios include the following:
- Threats to National Security and Law Enforcement
- Scenario 1 – Inciting Violence
- Scenario 2 – Producing False Evidence About Climate Change
- Scenario 3 – Deepfake Kidnapping
- Scenario 4 – Producing False Evidence in a Criminal Case
- Threats to commercial operations
- Scenario 1 – Corporate Sabotage
- Scenario 2 – Corporate Enhanced Social Engineering Attacks
- Scenario 3 – Financial Institution Social Engineering Attack
- Scenario 4 – Corporate Liability Concerns
- Scenario 5 – Stock Manipulation
- Threats to Society
- Scenario 1 – Cyber Bullying
- Scenario 2 – Deepfake Pornography
- Scenario 3 – Election Influence
- Scenario 4 – Child Predator Threat
How does Truuth mitigate the risk
Current mitigation tactics tend to focus on the development of technological solutions, primarily automated deepfake detection. However, as deepfakes progress and become more pervasive and ubiquitous, this single-minded approach will no longer be adequate, placing individuals and organizations on the defense and in a constant battle to catch up with the latest threat. This reactive approach would be both inefficient and needlessly risky.
There is no silver bullet to mitigate the risks of deepfake identities. At Truuth, we believe in a holistic approach to detection and mitigation which includes the following capabilities:
- Hashing of PII data to limit the source of deepfake creation
- Redaction of PII fields on images of ID documents
- Conversion of face images to irreversible vectors
- Matching of vectors and hashed PII to prior customers (and known fraudsters)
- Passwordless risk-based authentication including biometrics to verify the human (not just device)
- Account recovery that removes dependence on security questions and One Time Passcodes (OTPs)
- Binding of Verification of Identity (VOI) to user authentication
These solutions deliver step-change in identity verification across the entire customer lifecycle, from onboarding through every subsequent interaction.
If you would like more information, reach out to the Truuth team for a demo of our deepfake detection and risk-based MFA solutions.
Next article
Want to mitigate fraud? You need to integrate ID proofing, user authentication, and threat detection
Deepfakes are an emergent threat falling under the greater and more pervasive umbrella of synthetic identities. They utilize a form of artificial intelligence/machine learning (AI/ML).